Glencore’s Intercompany Reconciliation made easy with data-driven intelligence | Int4 Case Study
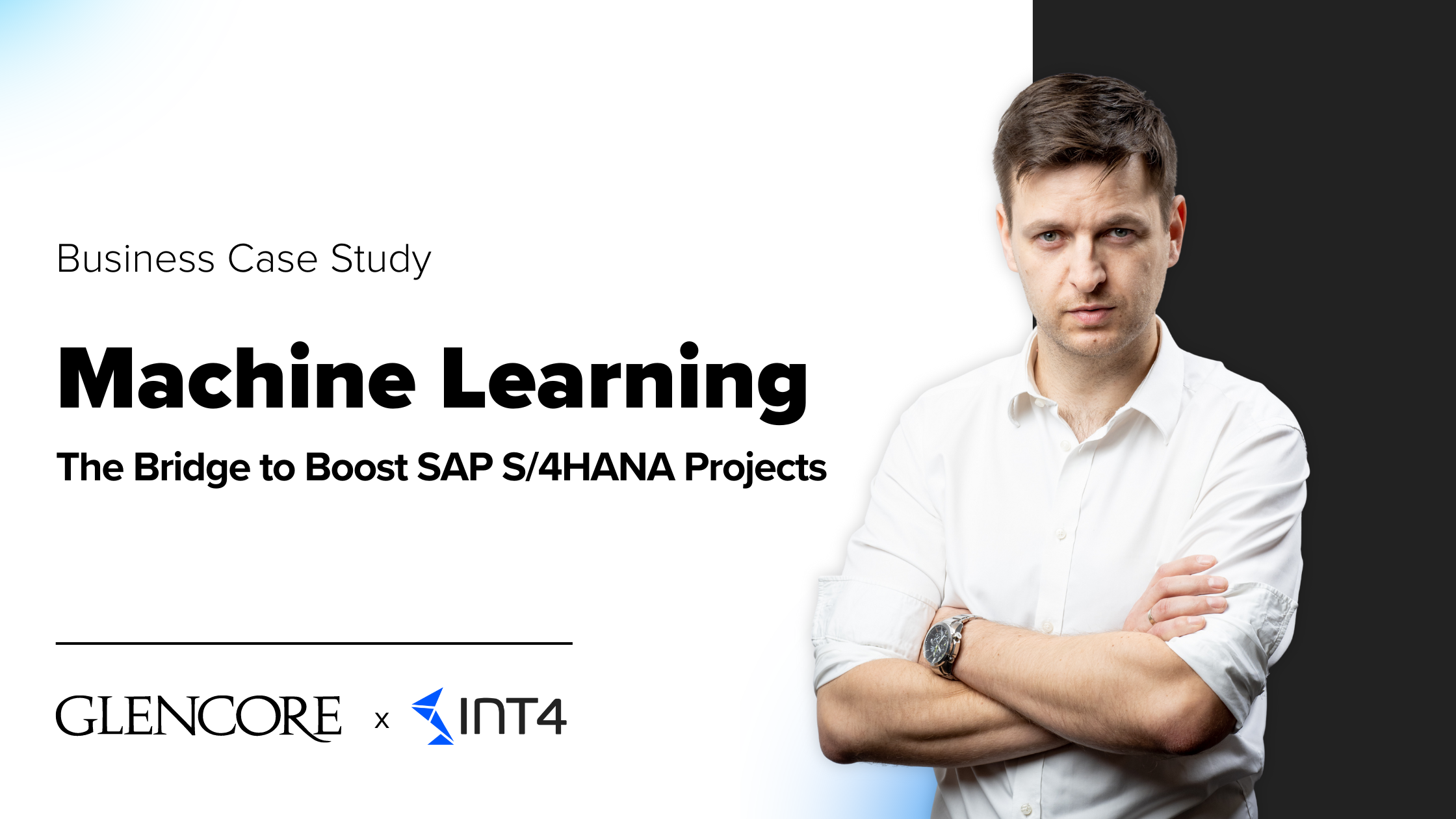
Business Summary: See what Maria Koletti says about the project results!
“Integrating data-driven intelligence into our intercompany reconciliation process has significantly enhanced our accounting operations. By leveraging machine learning to match transactions item-to-item, we have achieved unprecedented transparency, ensuring compliance and easing audits.
Not only has this boosted efficiency by streamlining reconciliation efforts, but also it has enabled continuous accounting, allowing us to proactively address discrepancies well before month-end closing.
Moreover, employing machine learning enables us to rely on dynamically determined matching rules, rather than static definitions, ensuring adaptability to evolving transactional patterns and complexities. This dynamic approach allows us to capture nuances and variations in data, ultimately leading to more accurate and robust intercompany reconciliation outcomes.
This transformation has truly empowered our finance team to operate with precision and agility.”
– Maria Koletti – Head of SAP Center of Excellence at Glencore
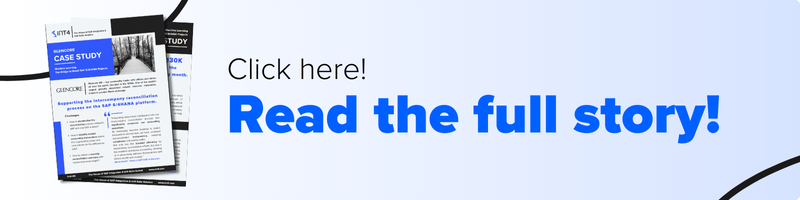
Customer Profile – about Glencore AG
Glencore AG is a top commodity trader with offices and mines all over the world, founded in the 1970s. One of the world’s largest globally diversified natural resource companies. Listed in London Stock exchange.
With more than 130 000 line items in the process every month, this case study is regarding Supporting the intercompany reconciliation process on the SAP S/4HANA platform.
About the project
Glencore teamed up with Int4 to simplify and speed up their intercompany reconciliation process using machine learning. This case study shows how they achieved better transparency, easier audits, and more efficient accounting. If you’re interested in improving financial operations and reducing manual work, keep reading to see how Glencore made it happen.
Challenges
Looking from a high level, during this project we wanted to answer three main questions:
- How to standardize the reconciliation process between SAP and non-SAP entities?
- How to identify related accounting transactions inside the organization group and concentrate on the differences only?
- How to deliver a monthly reconciliation summary with transaction-level insight?
Pain-points
- Different process approaches for every location.
- Reconciliation tasks are labor-intensive and spreadsheet-based.
- Manual, repetitive, and time-consuming procedures are error-prone.
- Reporting across all entities is inconsistent.
Solutions
Below, you will find the solutions that were implemented.
Machine Learning in SAP S/4HANA
The availability of machine learning libraries embedded in the SAP S/4HANA platform enabled a fresh design approach. Instead of implementing static rules, it was possible to provide an adaptive data-driven approach. The entire process was fully supported by a simple and straightforward UI built on SAP Fiori and AFO Workbooks.
The project’s goal was to provide a single solution for all types of entities (both internal and external). It was made possible through the introduction of a toolset capable of reconciling transactional data within the S/4 system, as well as postings from external sources, which are fed into the S/4 persistence layer via a unified user interface.
This helped to avoid time-consuming, spreadsheet-based reconciliation activities during period-end close. With a fully customized structure, scenarios can be reconciled between groups of entities (many to many). The approach, which is backed from start to finish by a consistent toolset, has the potential to promote transparency and collaboration among accounting teams.
Models are trained using 230 matching dimensions.
The Predictive Core
The automation of the procedure was enabled by implementation of a matching engine entirely based on the machine learning PAL library. The range of methods included (by default) in the SAP S/4HANA database enabled data-driven insight into the reconciliation process, allowing users to focus solely on uncommon circumstances.
The system’s advised interpretation could be validated and, if necessary, adjusted manually based on user comments. It made the entire process more adaptable and flexible in accordance with business requirements.
The generation of automatic groups for comparable accounting transactions saves time and work for the team by focusing their attention on unmatched items exclusively.
State of the art technologies
The entire solution was created using the most recent solution available in the SAP S/4 HANA platform.
Standard PAL libraries provide the matching engine’s capabilities and flexibility. The generated models are fully integrated with the ISLM framework. The reconciliation toolset was implemented in a simple and straightforward UI built on SAP Fiori.
Additionally, SAP S/4HANA embedded analytics enhanced the system with a reporting layer on AFO Workbooks. It provides users with insight into the process at several levels, including more general (for auditors) and detailed (if necessary).
Solution’s Benefits
The above described case is live in Glencore, a top commodity trader with offices and mines all over the world, founded in the 1970s. One of the world’s largest globally diversified natural resource companies. Listed in London Stock exchange.
Average matching level: 95%.
Gains
- Standardize reconciliation process across all business entities.
- Reduce pressure during period end closing.
- Allow accountants to focus on unmatched items.
- Improve reconciliation transparency and team cooperation.
- Integrate AI into your organization.
Utilize the power of Machine Learning in your Enterprise
By adopting Machine Learning in SAP S/4HANA, you can achieve remarkable efficiency, transparency and accuracy in your business processes.
If you’d like to learn more about how Int4 can support your enterprise in utilizing the modern SAP technologies, don’t hesitate to contact Michał Michalski – Int4 Partner & Head of Global Services.
E-mail: michal.michalski@int4.com
Popular tags
ABAP int4 INT4 IFTT Int4Interview S/4HANA SAP AIF SAP CPI sap integration